AI in banking – soon essential to succeed?
The financial sector is one of the most data-intensive sectors of the global economy. The ability to make effective use of these vast amounts of data promises significant competitive advantage. But what are the prerequisites for realising the full potential of this mountain of data? Sonja Huclova and Stefan Fröhlich provide the answers.
Interview with Sonja Huclova and Stefan Fröhlich
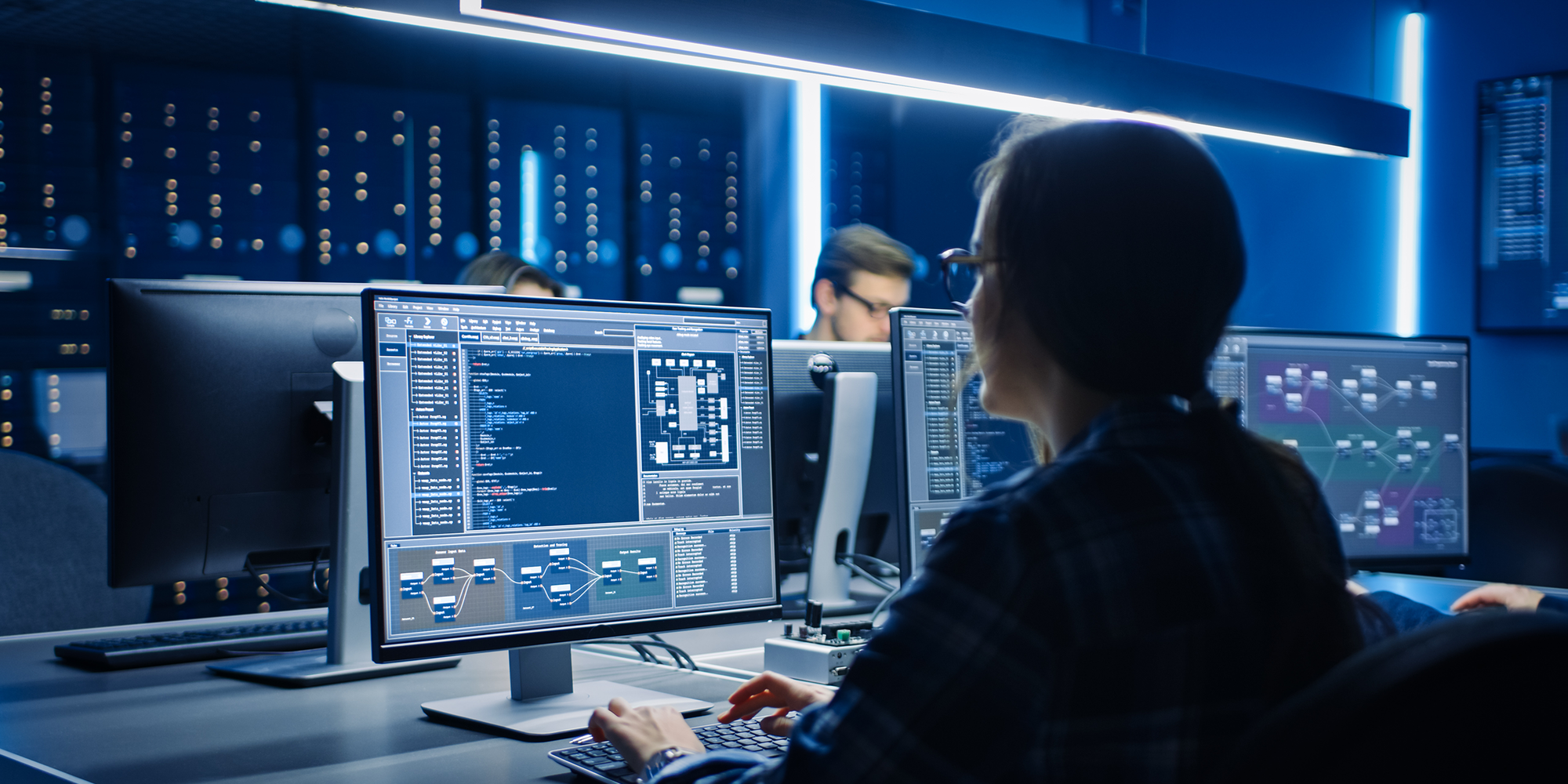
Stefan, what data is available in the financial sector already and how can it be used?
The data ranges from customer information and transaction data to market data, macroeconomic indicators and social media. The integration and analysis of this data can help banks and financial service providers to gain deeper insights into customer behaviour, create data-based predictions, better manage risks and identify market opportunities more efficiently.
Much hope is pinned on the use of Artificial Intelligence (AI). What specific areas of application are there in asset management?
Hundreds of key figures are available for each company, which can influence stock market prices. By skilfully processing these data volumes, machine learning models can independently recognise patterns and uncover hidden correlations between company key figures and future returns. In this way, daily forecasts can be derived for the price development of shares worldwide. These predictions can then be implemented in funds and mandates using a sophisticated portfolio construction process. Zürcher Kantonalbank Asset Management has had a tried-and-tested machine learning algorithm for stock selection for several years and uses it successfully.
Large Language Models (LLM) are particularly in the spotlight. Where are they used in asset management?
One field of application is the automated processing of news, company reports and conference calls. Sentiment indicators can be derived for each company on a daily basis. These show whether news reflects a positive or negative mood. These sentiment indicators can be used as an additional source of information to make well-founded investment decisions. LLM also support portfolio managers in their favourite discipline, namely picking out price-relevant information from the thicket of data. Specially trained GPT (Generative Pre-trained Transformers) can help to summarise analyst reports and extract the relevant information.
To what extent is the profession of portfolio managers and analysts at risk?
It will still be several years before artificial intelligence can emulate human intelligence in all its facets, such as reasoning, visual perception or motor skills. Nevertheless, AI already offers considerable advantages in some areas. For example, it can analyse huge amounts of data in a very short time and independently recognise patterns that often remain invisible to humans. The key question is: how can we use AI skillfully to support our work, make better decisions and increase our efficiency?
Sonja Huclova, Head AI Specialist Centre
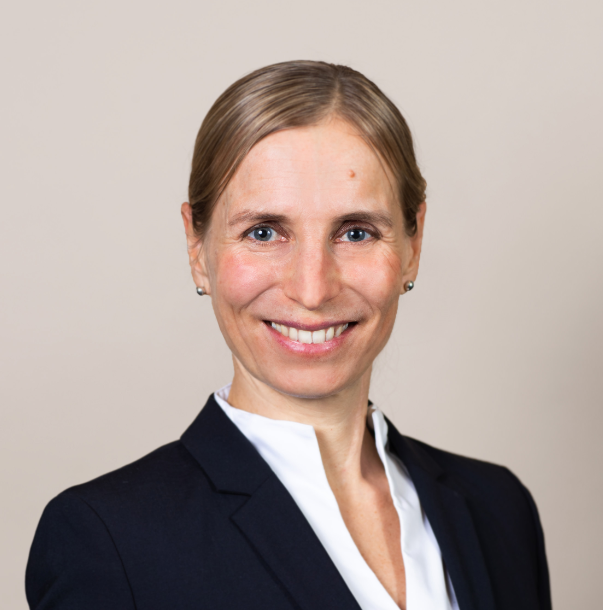
Sonja holds a Master's degree in Chemistry from the University of Bern, a PhD in Electrical Engineering from ETH Zurich and a CAS in Big Data from BFH. She has worked for a transport company as a systems engineer in the context of safety and security, as a solutions specialist in the field of test simulation and test management, as well as an expert on IT governance topics for an IT service provider. She has been part of the data science team at ZKB since 2020 and leads the AI technology group.
Stefan Fröhlich, Portfolio Manager Systematic Equities
Stefan is an equity fund manager and has developed various systematic strategies. In addition to multi-factor models and trend-based behavioural finance approaches, he has been intensively involved with ai and its application in asset management for ten years. Previously, he worked as a fund manager for systematic and fundamentally managed equity funds and an equity strategist at GAM and Julius Bär. Stefan studied environmental engineering at ETH Zurich and holds a Master in Business Administration (MBA) from ETH Zurich. He is a certified CIIA (AZEK).
Let's come back to banking. Sonja, can AI also be used in the interest business, for example in lending?
Credit checks are tending to become more complex. One of the reasons for this is the increasing regulatory requirements placed on institutions. Increasing digitalisation and computing power are also contributing to this, as more and more data points and sources can potentially be included in the credit check. Although large volumes of data enable more precise analyses, they also require more complex models and generally the use of AI algorithms. AI models should ultimately serve to provide data-based support for the advisor's final decision.
And what about estimates of real estate prices?
There are numerous rule-based models that incorporate many price-sensitive influencing factors. These include factors such as location, condition, geographical environment and energy efficiency. In addition, there are external circumstances such as interest rates, market trends or regulatory requirements such as rezoning. Similar to lending, the price estimation process is also becoming increasingly complex - with corresponding potential for AI.
Why does it make sense for banks to set up an AI centre – and what should it look like?
The optimal setup for the implementation of AI in banks includes several aspects. Put simply, it is an interplay of infrastructure, data and people. People should have the best possible conditions for developing, using and mastering the technology. However, there are a few things to consider.
What would that be?
Firstly, a suitable infrastructure is required. It enables access to the sometimes very large and usually distributed data. This is absolutely crucial, because the added value that AI can bring as a method lies precisely in the simultaneous processing of a wide variety of data. In addition to integration capability, functionalities for optimising the creation of code are another important element of the infrastructure. Efficient data processing is only made possible by means of code and specific functionalities. Finally, an optimal infrastructure includes sufficient computing power for the resource-hungry AI models.
So not all data is the same?
Clean data is essential, as AI also scales inadequate data quality, which is reflected accordingly in the results. Overall, it should support both centralised topics and enable implementation in the bank's specialist departments (see illustration below).
Is a bank well positioned with this setup?
The existence of a central AI centre is of course an advantage. It supports everyone involved in the AI process. From consulting to enabling and awareness for the entire bank, it also makes sense to help shape key fundamental topics. The great added value lies in helping to harmonise overarching topics such as data, architecture, infrastructure and governance. Otherwise, there is a risk that uncontrolled redundancies and technical debt will arise, which will be very costly financially in the long term at the latest.
Is there a need for a guiding hand?
This may sound a little too rigorous – but yes, a fragmented landscape makes it difficult to provide information to regulators. Like the infrastructure, the AI specialist unit should optimally support existing decentralised implementation organisations and work in close cooperation with an implementation team that implements orders for business areas without this special expertise. The effective form of such a specialised unit will vary from bank to bank- However, dealing with the topics mentioned is generally valid.